Reasoning Models May Hit a Wall Sooner Than Expected
While OpenAI has dramatically ramped up computing for RL—reportedly using 10 times more resources for o3 than for its predecessor— there's a limit to how much this approach can scale.
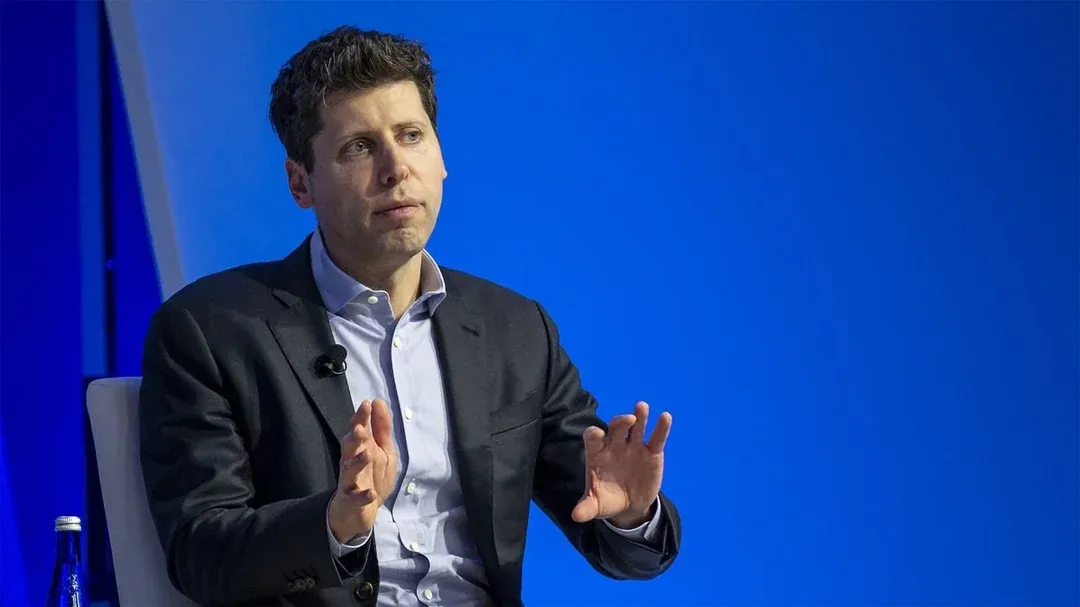
Performance gains from reasoning-based AI models—like OpenAI’s o3—may soon plateau, possibly within a year, according to a new study.
These models, which excel in tasks like math and programming, benefit from reinforcement learning (RL), a technique that improves problem-solving through feedback.
While OpenAI has dramatically ramped up computing for RL—reportedly using 10 times more resources for o3 than for its predecessor— there's a limit to how much this approach can scale.
"If reasoning training continues to scale at 10× every few months, in line with the jump from o1 to o3, it will reach the frontier of total training compute before long, perhaps within a year. At that point, the scaling rate will slow and converge with the overall growth rate in training compute of ~4× per year. Progress in reasoning models may slow down after this point as well," a new analysis by nonprofit research institute Epoch AI reads.
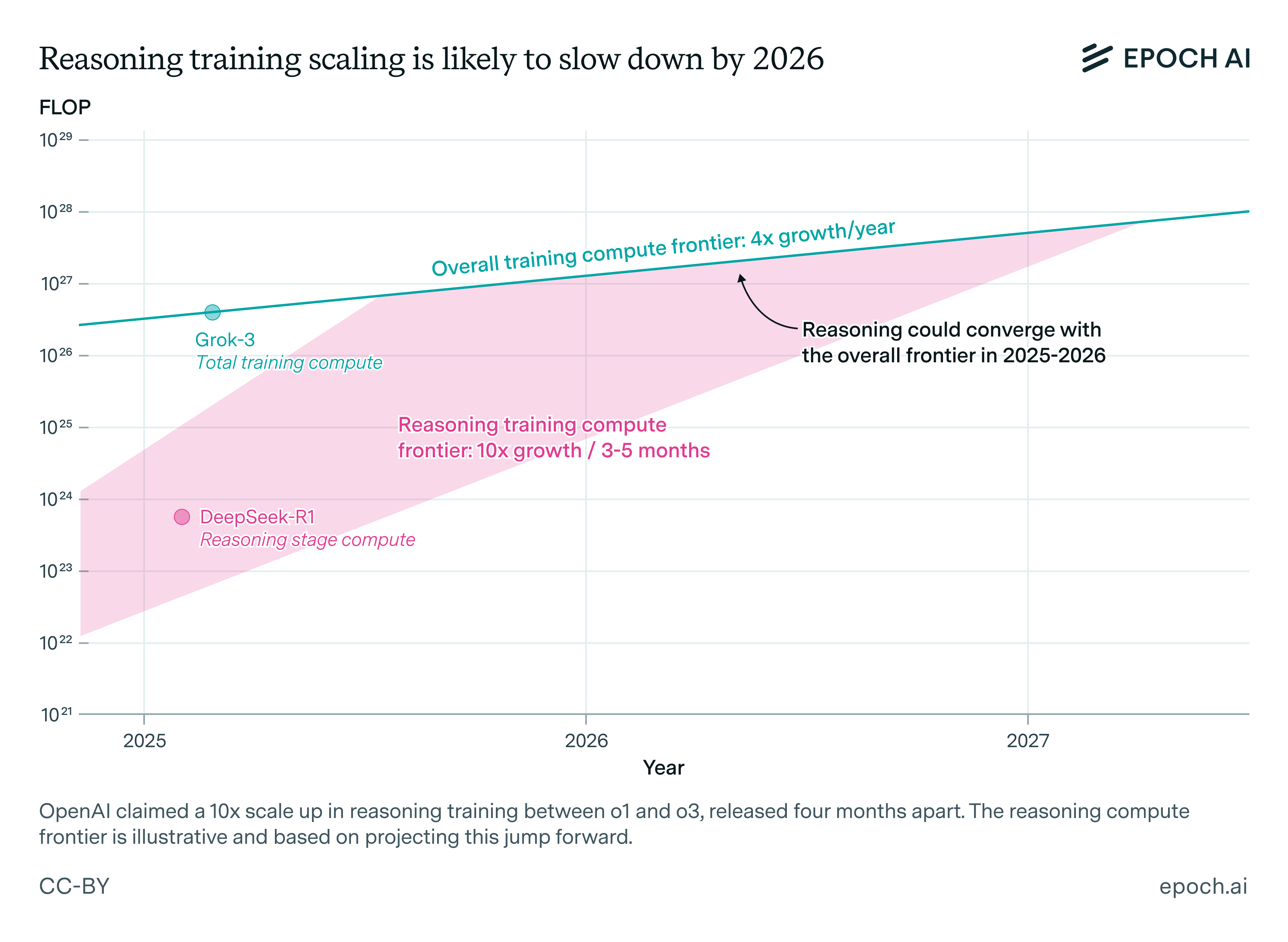
Epoch also notes that while traditional training yields gains that quadruple annually, RL-driven improvements have been growing tenfold every 3–5 months.
That trend may taper off by 2026, as the frontier of reasoning models converges with broader AI performance ceilings.
High costs and technical complexity could further hinder progress, raising concerns in an industry betting big on reasoning AI despite its flaws and high operational costs.
Last month, OpenAI released two new models – o3 and o4 mini– yet, in a technical paper, they admitted that these models hallucinate more than their predecessors.
In fact, on OpenAI’s PersonQA benchmark, o3 hallucinated on 33% of queries — more than double the rate of o1 and o3-mini. O4-mini performed even worse, hallucinating 48% of the time.
Once again, these developments raise questions about the hype surrounding generative AI, which often promises rapid, exponential progress but may be approaching technical and economic limits that could slow future breakthroughs.